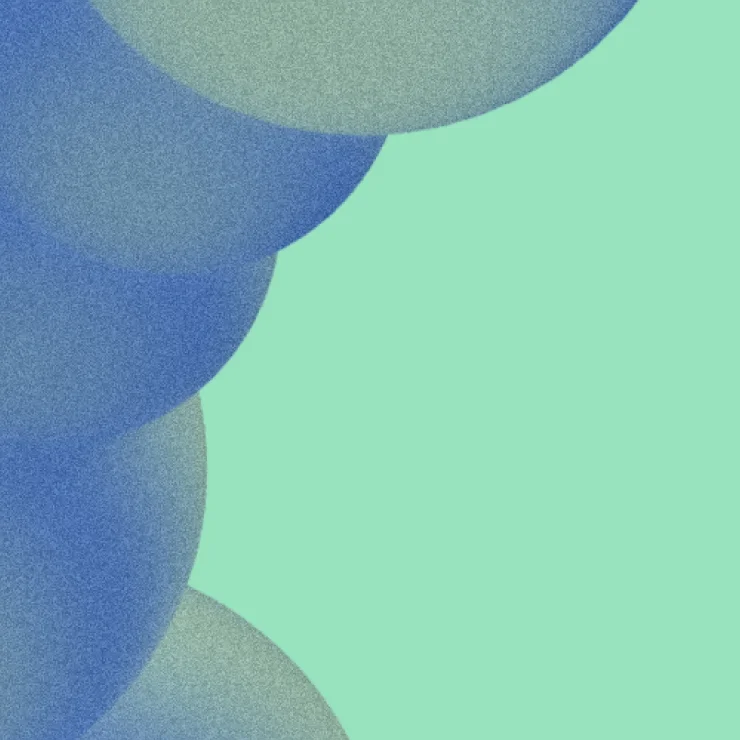
ALFRED Platform
WhiteLab Genomics’ proprietary AI platform accelerates target discovery and drug design for genomic medicine. Combining advanced algorithms, curated databases, and cutting-edge computational biology, we optimize therapeutic candidates for safety and efficacy across diverse modalities such as AAV, lentivirus, and lipid nanoparticles.
Overview
Key Features
By integrating biomarker mapping, receptor-ligand prediction, and transcriptomic analysis, our platform streamlines genomic medicine development, reducing risks and accelerating time-to-market.
Our platform
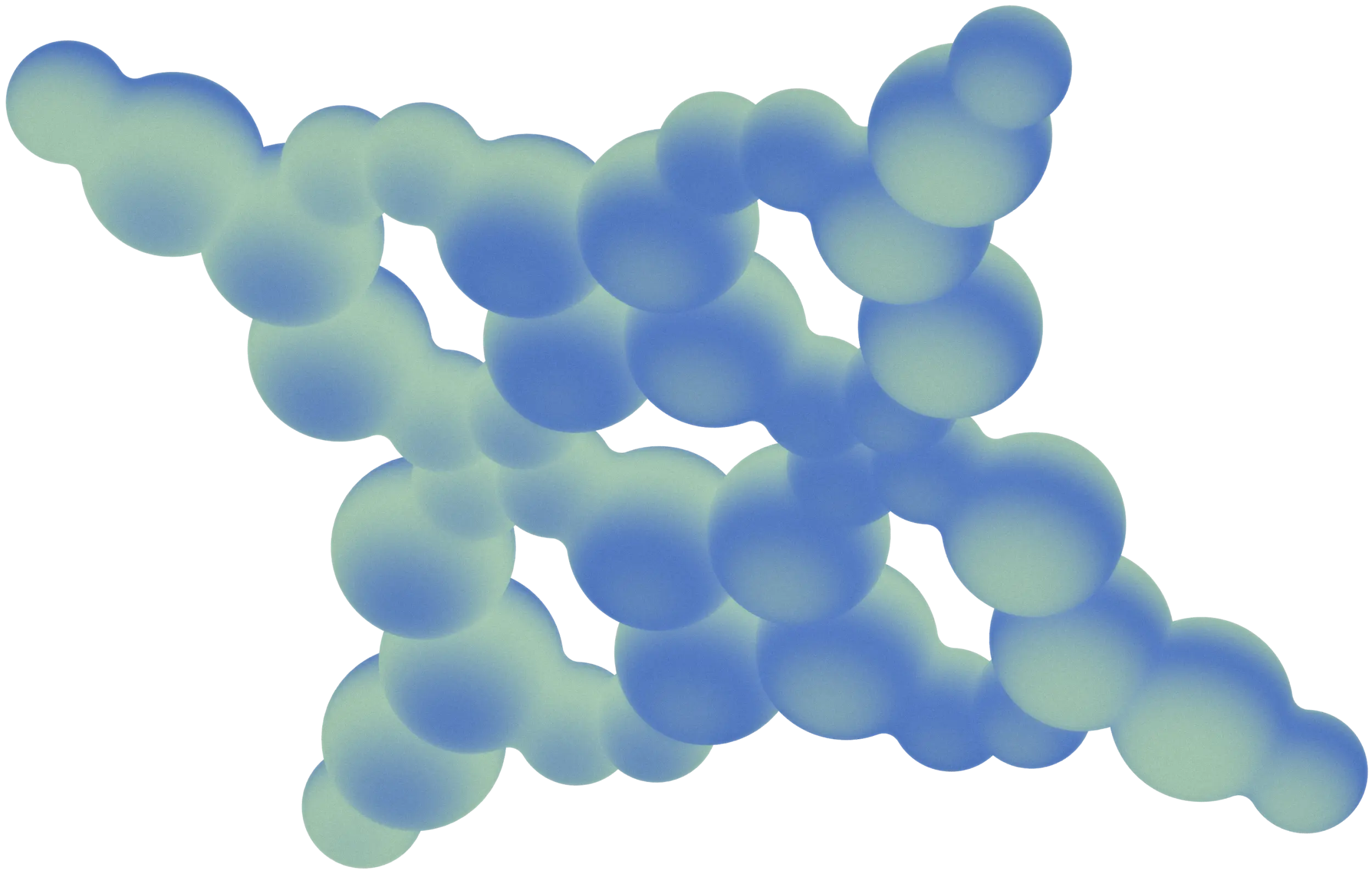
Proprietary data curation to fuel breakthroughs
- Untapped public and proprietary data for Genomic Medicine
- WhiteLab works with top academic centers to curate, enrich, and generate data that is AI-ready
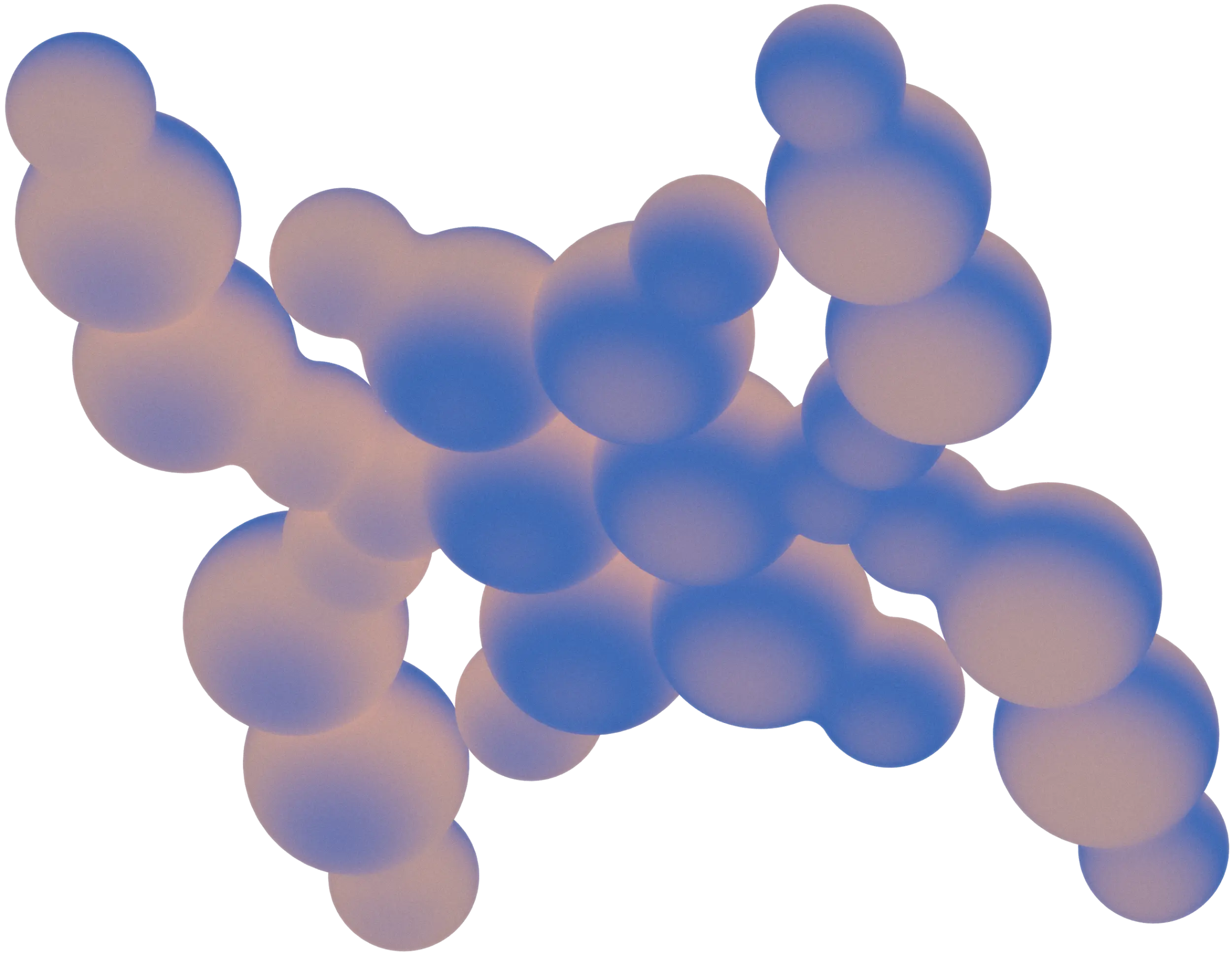
Computational biology platform for Bio Asset Discovery
- Comprehensive Biomarker Cellular and Tissue Atlas
- AI-Based Therapeutic Target and Identification of Target Receptor
- Payload design and optimization
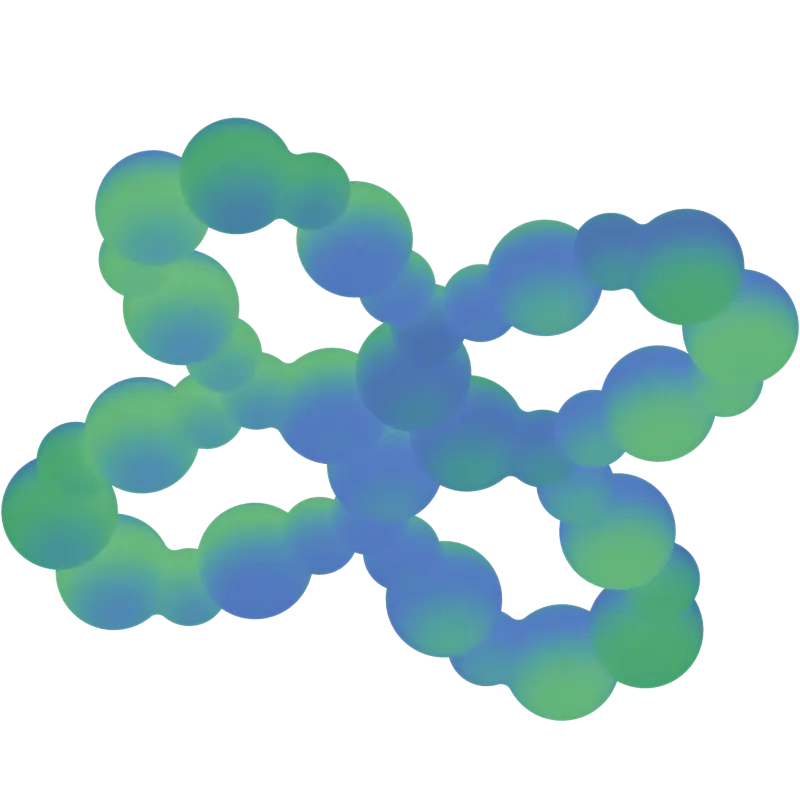
Structural biology database
- Unlocking untapped public and proprietary data (peptide, protein, vectors...)
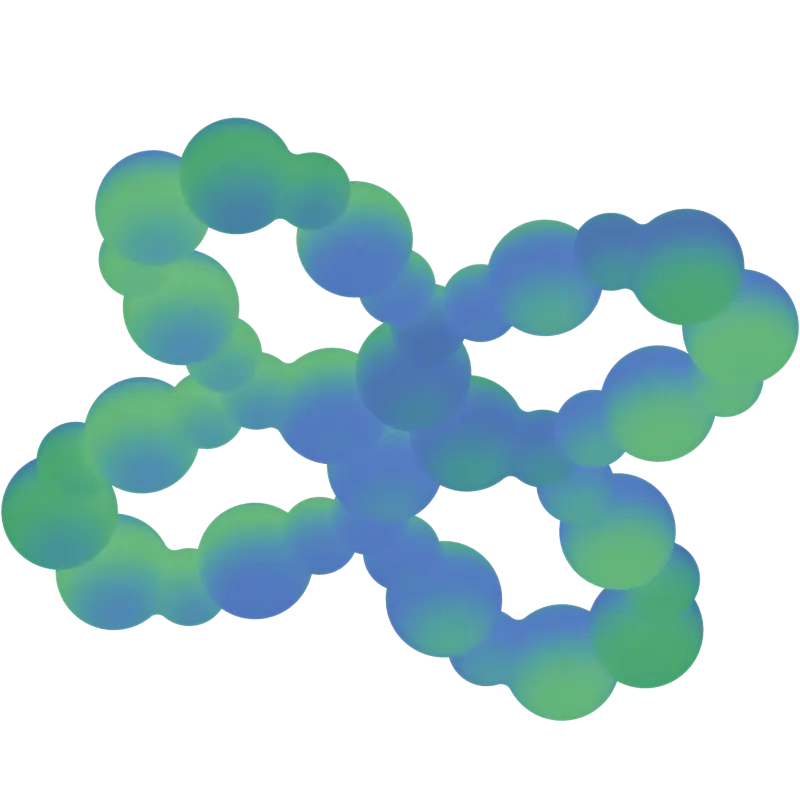
Structural biology insights for precise protein and vector engineering
- Affinity prediction: Identify, assess and optimize ligands binding therapeutical targets
- Combining AI algorithms with precise modelling for rational molecular design
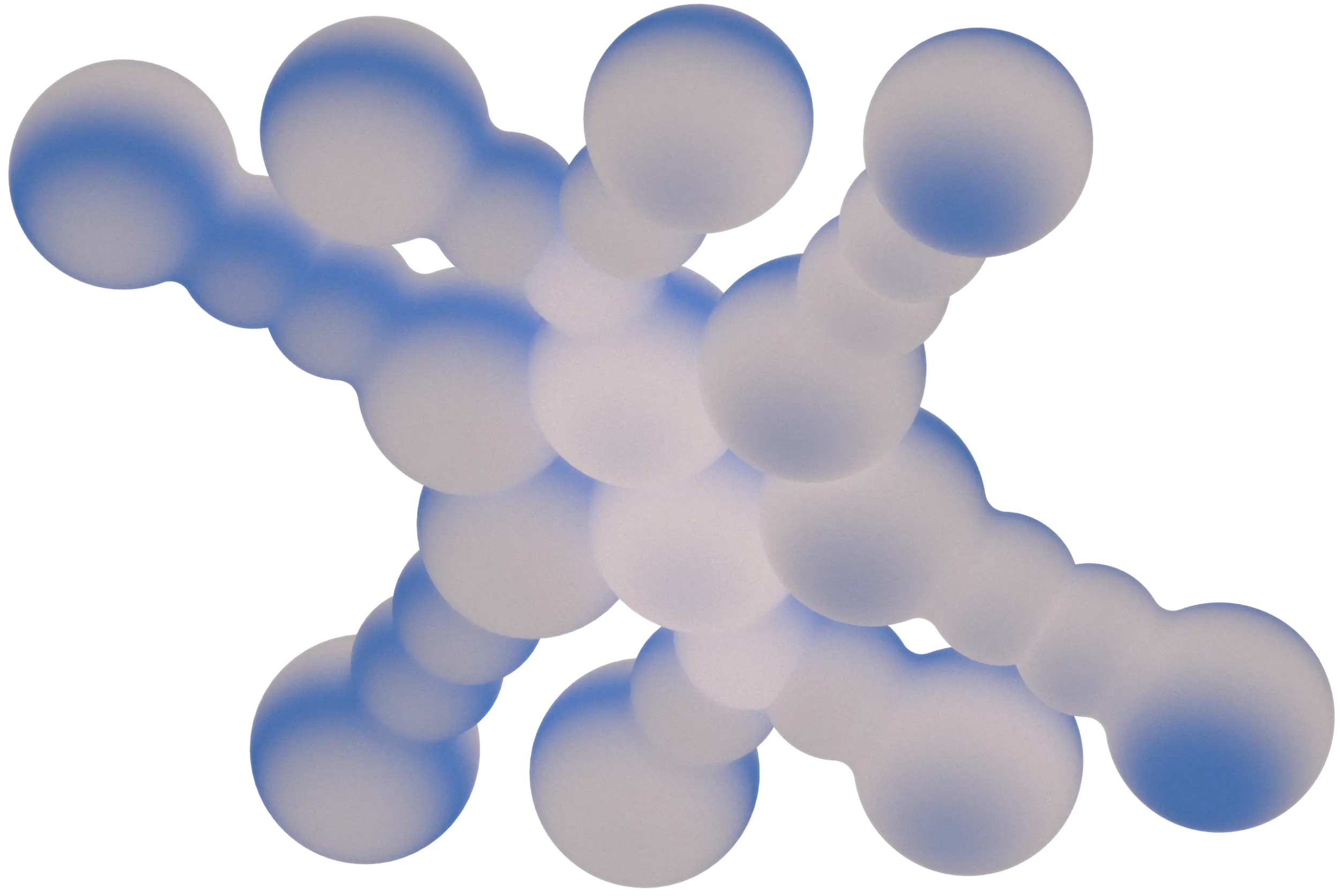
Data science toolbox for bio-optimization and prediction
- ML/GenAI approaches for Genomic Medicine
- AI approach for predictive modeling of Genomic Medicine target identification and design
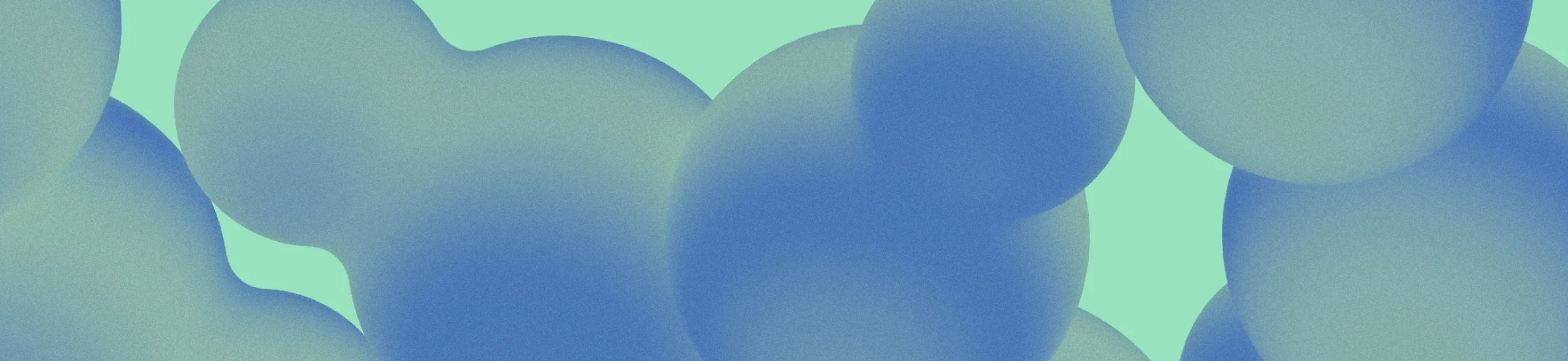
Technologies
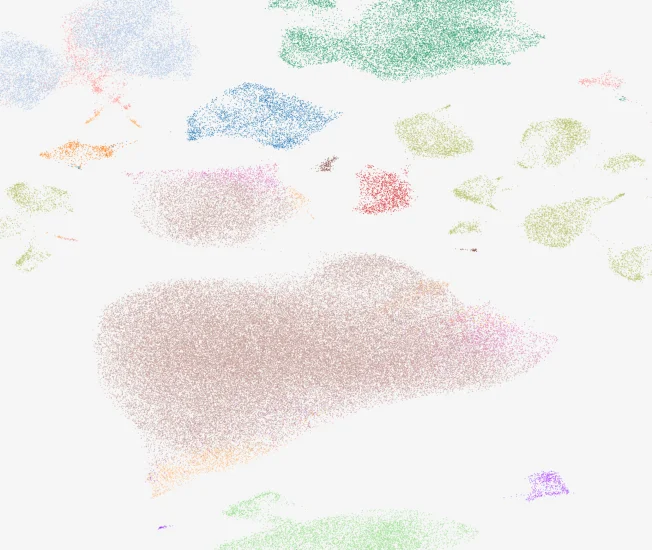
Comprehensive Biomarker Mapping
Our biomarker atlas includes 1,000+ datasets and 850+ cell types, providing precision for gene and protein targeting. Combining RNA sequencing, protein analysis, and ontology mapping, we deliver unmatched specificity and localization for receptor identification.
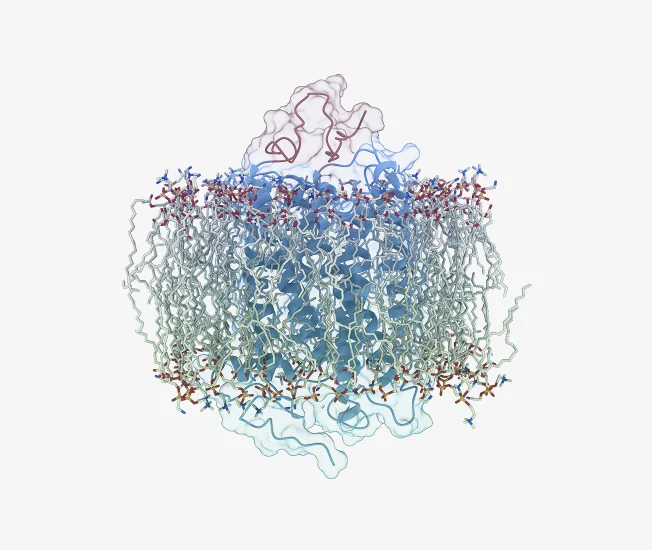
Cell Surface Protein Identification
Our advanced deep learning method predicts whether a protein is located at the cell surface based solely on its amino acid sequence. Leveraging the highly curated UniProt dataset, our model cross-checks its predictions with high-confidence subcellular localization data from the subcellular localization database.
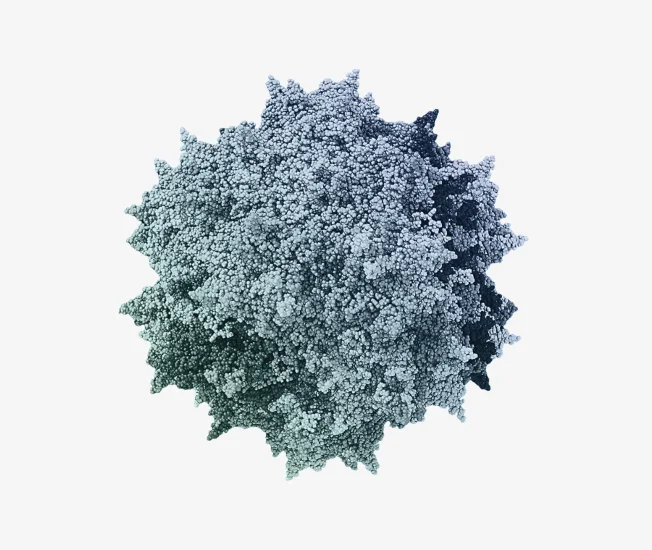
AAV Capsid Engineering
Protein Language Models and Reinforcement Learning are applied to design AAV capsids with enhanced safety and efficacy. By engineering novel amino acid sequences, we overcome challenges like immune evasion and tissue-specific targeting, driving innovation in gene therapy.