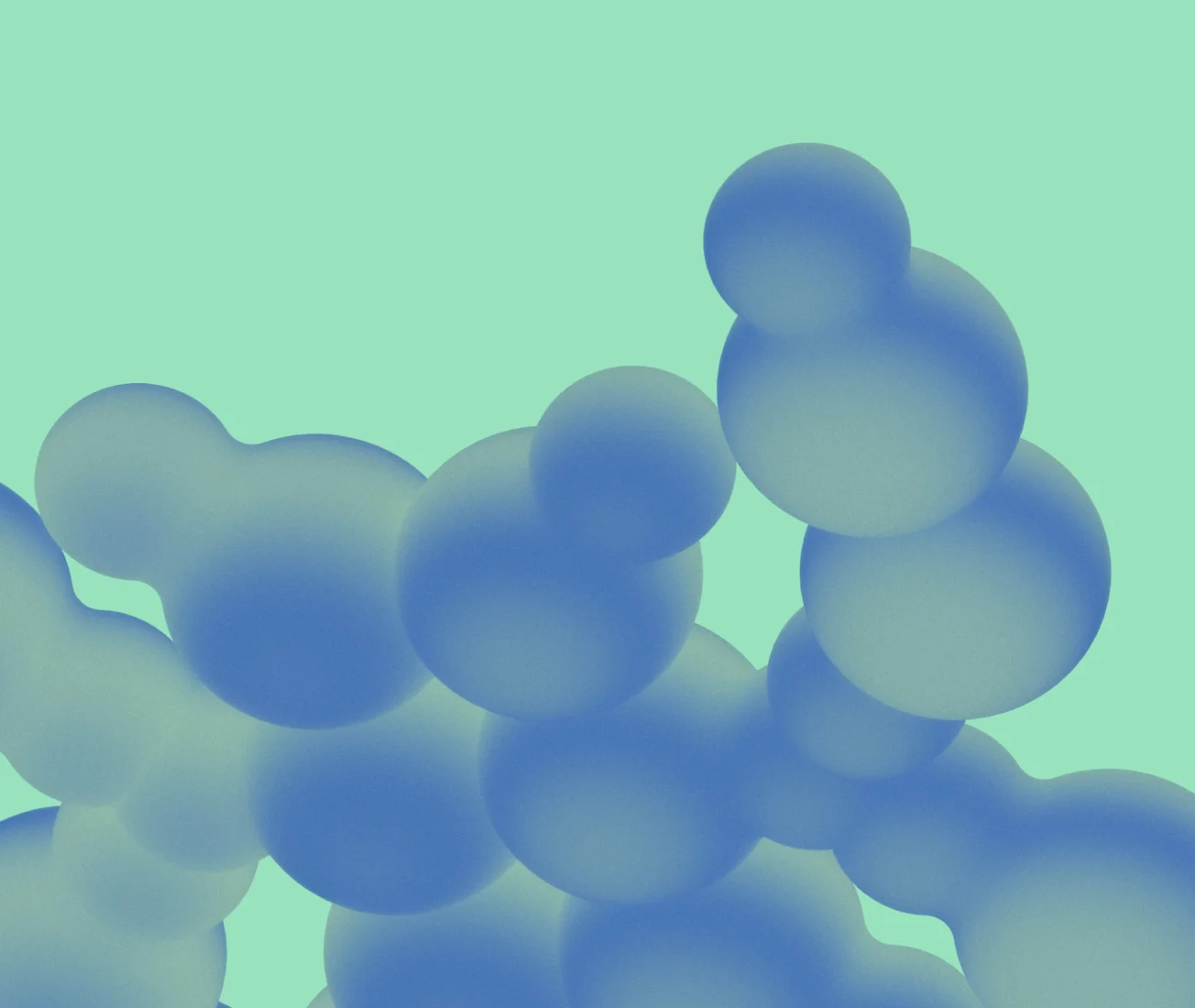
Innovative Approach to AAV Capsid Design
A computational approach that leverages Protein Language Models (pLMs) and Reinforcement Learning (RL) to engineer AAV (Adeno-Associated Virus) capsids. By focusing on permutations within the variable region VIII, our model generates novel amino acid sequences aimed at enhancing therapeutic efficacy and safety. This innovative approach addresses key challenges in gene therapy, such as immune evasion, tissue-specific targeting, and transduction efficiency.
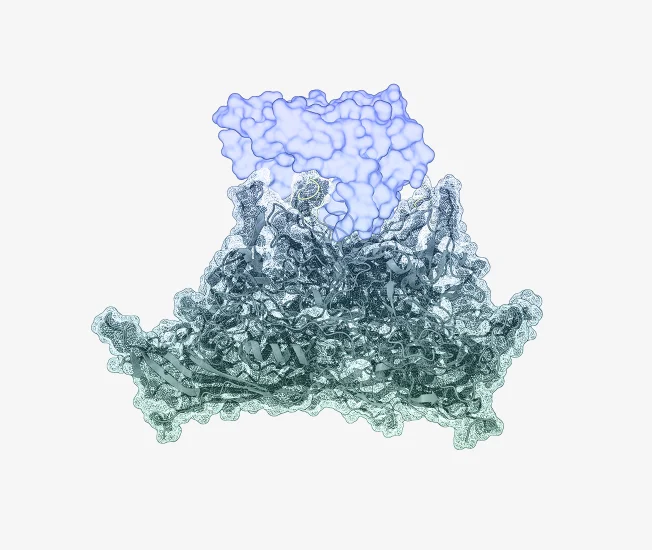
Integration of Protein Language Models and Reinforcement Learning
Utilizing the fine-tuned ESM-2 Protein Language Model, we accurately encode amino acid sequences, drawing on extensive functional and structural protein data. The integration of RL allows for the iterative refinement of these sequences, optimizing phenotypic traits and structural viability. This dual strategy enables exploration of a broader sequence space, fostering the development of manufacturable AAV variants with improved therapeutic potential.
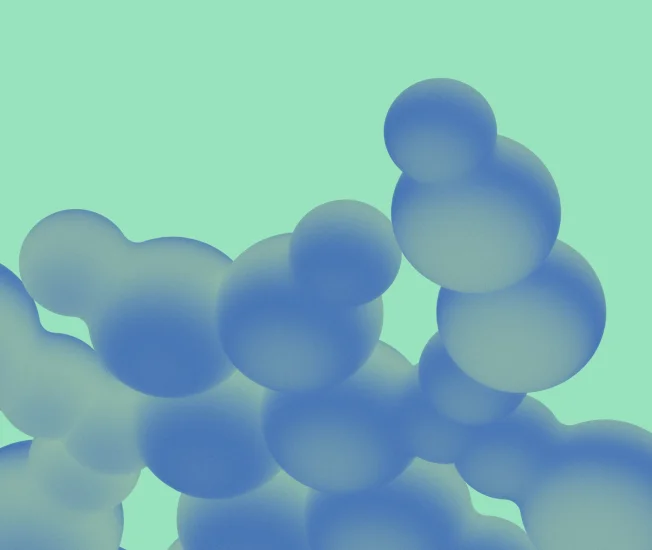
Performance and Potential Impact
The model applies a policy over four actions—deletion, addition, substitution, and inversion—rewarding viable sequence generation. Preliminary results suggest that this AI-driven methodology can preserve or enhance the desired phenotypic characteristics of AAV capsids, which will be validated through experimental research.
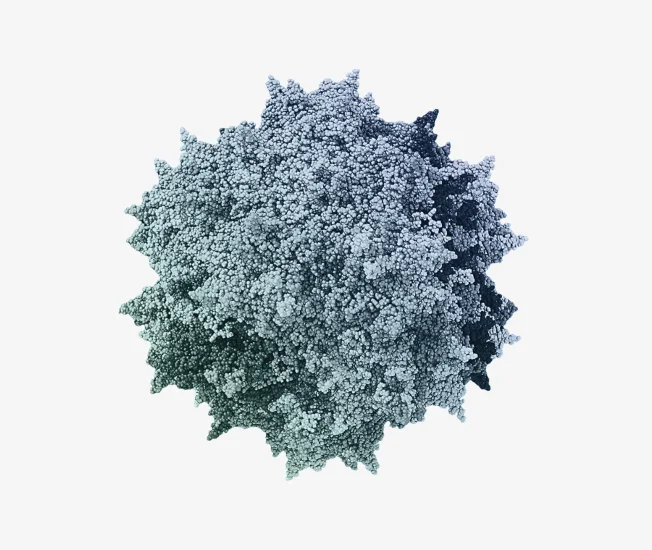
Advancing Therapeutic Interventions
This research underscores the advantages of combining advanced computational techniques in the design of gene therapy vectors, enhancing the precision and efficacy of therapeutic interventions. Our findings promise to advance the field of gene therapy by providing more targeted and efficient solutions.