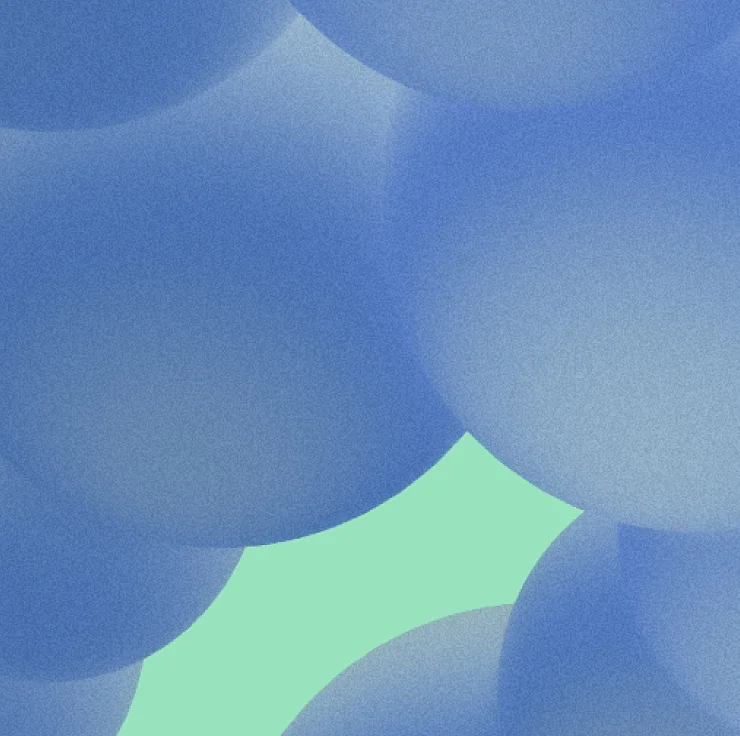
WhiteLab Genomics to Showcase AI-Driven Outcomes Advancing the Future of Genomic Medicine at ASGCT 2025
Whitelab Genomics, a techbio company specializing in artificial intelligence to accelerate the development of genomic medicines, announces the presentation of four research posters at the upcoming 28th Annual Congress of the American Society of Gene & Cell Therapy (ASGCT), taking place May 13-17th, 2025 in New Orleans, USA.
Enhancing AAV Gene Therapy Targeting Retinal Diseases with AI-Guided Rational Design
AMA1842 - Wednesday, May 14, 5:30PM - 7:00PM
Authors: A.Barberis, B. Dafniet, T. Van Meter, U. Ferrari, P. El Darazi, D. Serillon, D. Dalkara, J. Cottineau
WhiteLab Genomics, Future4Care, 8 rue Jean Antoine de Baïf, 75013 Paris, France
Institut de la Vision, Paris, France
Gene therapy using adeno-associated viral vectors (AAVs) has been proven to be clinically effective for the treatment of retinal dystrophies, with most mutations residing in the outermost retinal cells. To target these cells, viral vectors need to cross the inner limiting membrane, and inner retinal neurons making them more difficult to target via this injection route. Current viral vectors are mostly injected into the subretinal space to target these cells, albeit with the efficacy being limited to the bleb region. To address these challenges, we developed AI-guided, rationally designed approaches to engineer AAV2-derived vectors for ocular gene therapy, enabling low dose wider spread human retinal transduction via subretinal injection. We applied machine learning approaches to single-cell RNA-sequencing data and protein databases to identify receptors of interest for AAV targeting into human photoreceptors. We started with the identification of human photoreceptor-specific markers using our proprietary single-cell Atlas and analytical platform. To quantify their specificity to retina, we computed the average expression levels of these markers as well as their percentage of expression at the photoreceptors surface compared to other retinal cell types grouped together. We also computed key metrics such as localization information about associated proteins. Additionally, we evaluated their involvement in both immune and endocytosis pathways. To ensure cross-species relevance in experimental models for pre-clinical studies, we curated single-cell RNA-seq data from pig, performing quality control and cell annotation using automated and manual approaches to identify cell-specific markers in the pig retina. We then explored the conservation of these markers with human. Once markers expressed at the surface membrane were identified, computational structural biology approaches were performed for target validation. We assessed both the presence of already existing 3D structures and the capabilities of prediction tools to accurately reconstruct them in 3D when none was available. The presence of an interactor in the resolved structure was of importance to consider a marker as a potential candidate using our rational-guided approach. For the final step of our target validation approach, we performed analysis of structural conservation of the interacting site, when known, between human and pig. This allowed for a more effective cross-species AAV design and led us to the selection of one promising target, maximizing all our criteria for the design of novel viral vectors against retinal diseases. The next step will be the creation of optimized AAV libraries by inserting peptides targeting the receptor in the AAV2 vector sequence. Efficient delivery remains a major challenge in the development of gene therapies for retinal diseases. Our comprehensive approach integrating AI-driven rational design, cross-species marker validation, and computational structural biology helps to overcome this current limitation. By identifying conserved, surface-exposed targets, we have laid the foundation for the development of optimized AAV vectors with enhanced retinal transduction capabilities. These advancements bring us closer to addressing a critical unmet need for more effective and targeted treatments for hereditary retinal diseases.
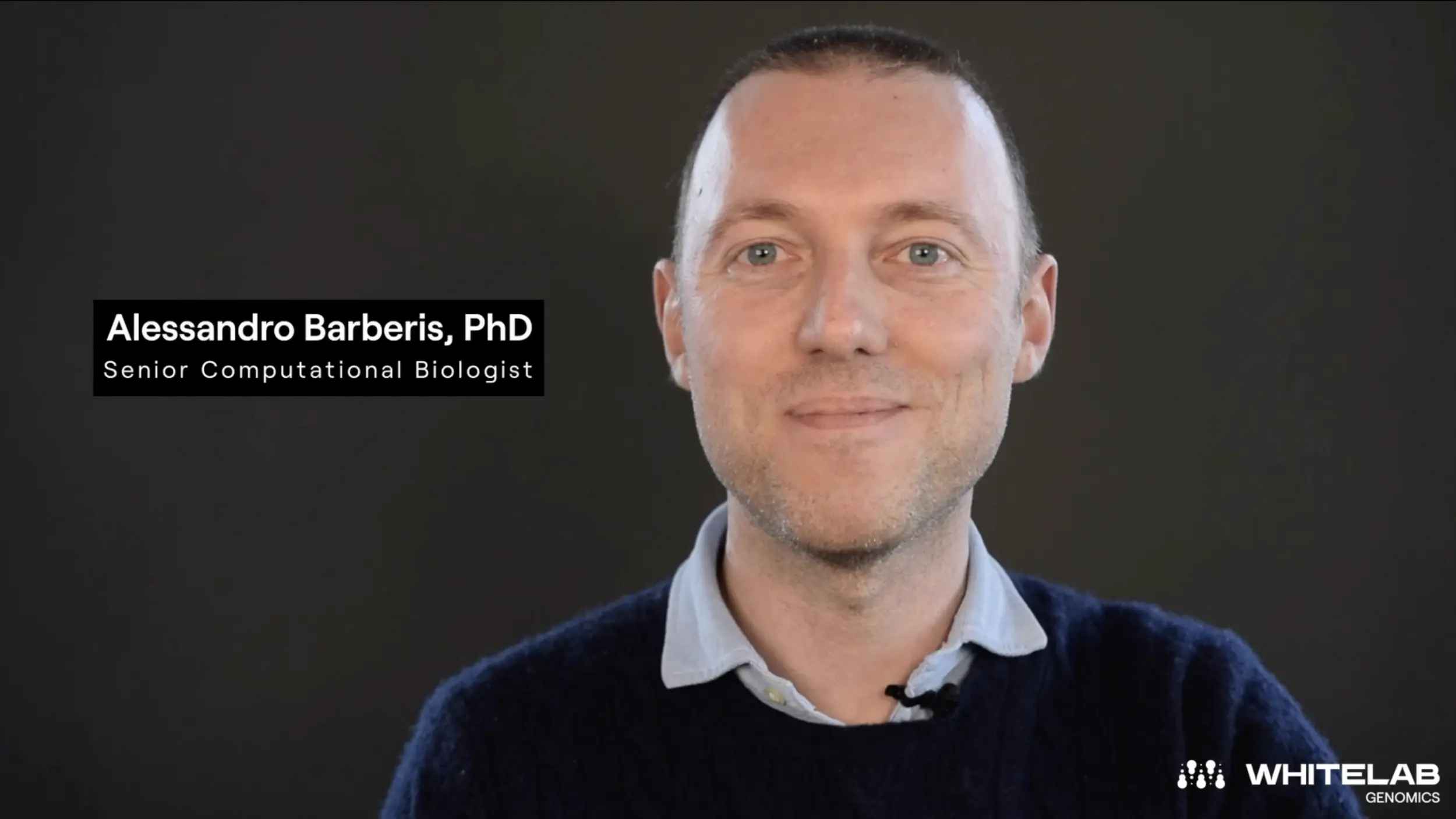
Novel In Silico Generation of a Synthetic Peptide Library Derived from Biological Complexes for Protein and Vector Engineering
AMA1560 - Thursday, May 15, 5:30PM-7:00PM
Authors: C.Alliot, B.Dafniet, Y.Habtoun, C.Colas, J.Maes, D. DelBourgo, J.Cottineau, D.Serillon
WhiteLab Genomics FUTURE4CARE, 8 rue Jean Antoine de Baif, 75013 Paris, France
The generation of chemical libraries, specifically peptide libraries, has been an ongoing topic for the past decades. Peptide design is actively being researched due to their specific properties (stability, binding...) both as a therapeutic agent and, in the cell and gene therapy field (C&G), for their targeting properties. They can be combined with various viral or non-viral vectors (e.g., adeno-associated viruses, nanoparticles...) as they have a diverse set of association possible ranging from, but not limited to, peptide conjugation as well as insertion in both linear and cyclized conformations. A common strategy for designing peptide libraries involves using a random approach, which typically leads to a large volume of candidates, with a high proportion of structurally and biologically irrelevant sequences, driving up both cost and time in identifying relevant binders. Despite the rapidly evolving field, the concept of in silico peptide library, especially based on rational biological complexes, represents an important breakthrough, yet remains largely unexplored due to its complex nature. WhiteLab Genomics is presenting a novel in silico approach, stemming from structurally resolved experimental data from protein-peptide complexes. The objective is to create a peptide library specifically tailored in a vector engineering context for gene therapy that can also be used in the discovery of pertinent motifs for new therapeutic peptides. It is based on biologically active motifs that, when combined with WhiteLab Genomics’ rational-guided approach, can enhance both in silico discovery and evaluation of new hits against a receptor of interest reducing the risk of having biologically irrelevant peptides. It led to the generation of more than 300 thousand unique sequences, ranging from 5 to 12-mer peptides, reconstructed in 3D, in four initial conformations, namely helix, linear, cyclized and beta-sheet, forming a total library of more than 1.2 million unique structures. This allows expanding synthetically the chemical space of available peptides by exploring a highly diverse conformational space. Every newly generated structure is mapped and characterized with sequence and structural descriptors. To significantly improve the success rate of our approach, we also developed a subset called the Diversity library composed of peptides representative of key properties. It enhances both the search for efficient therapeutic screening and improves the engineering of a vector of choice by allowing us to explore a large chemical space while extracting a minimal number of peptides sharing similar properties. Finally, the generated properties in both these libraries will also be useful in the development of tailored machine-learning or artificial intelligence models, paving the way for new and better solutions in the C&G therapy field.
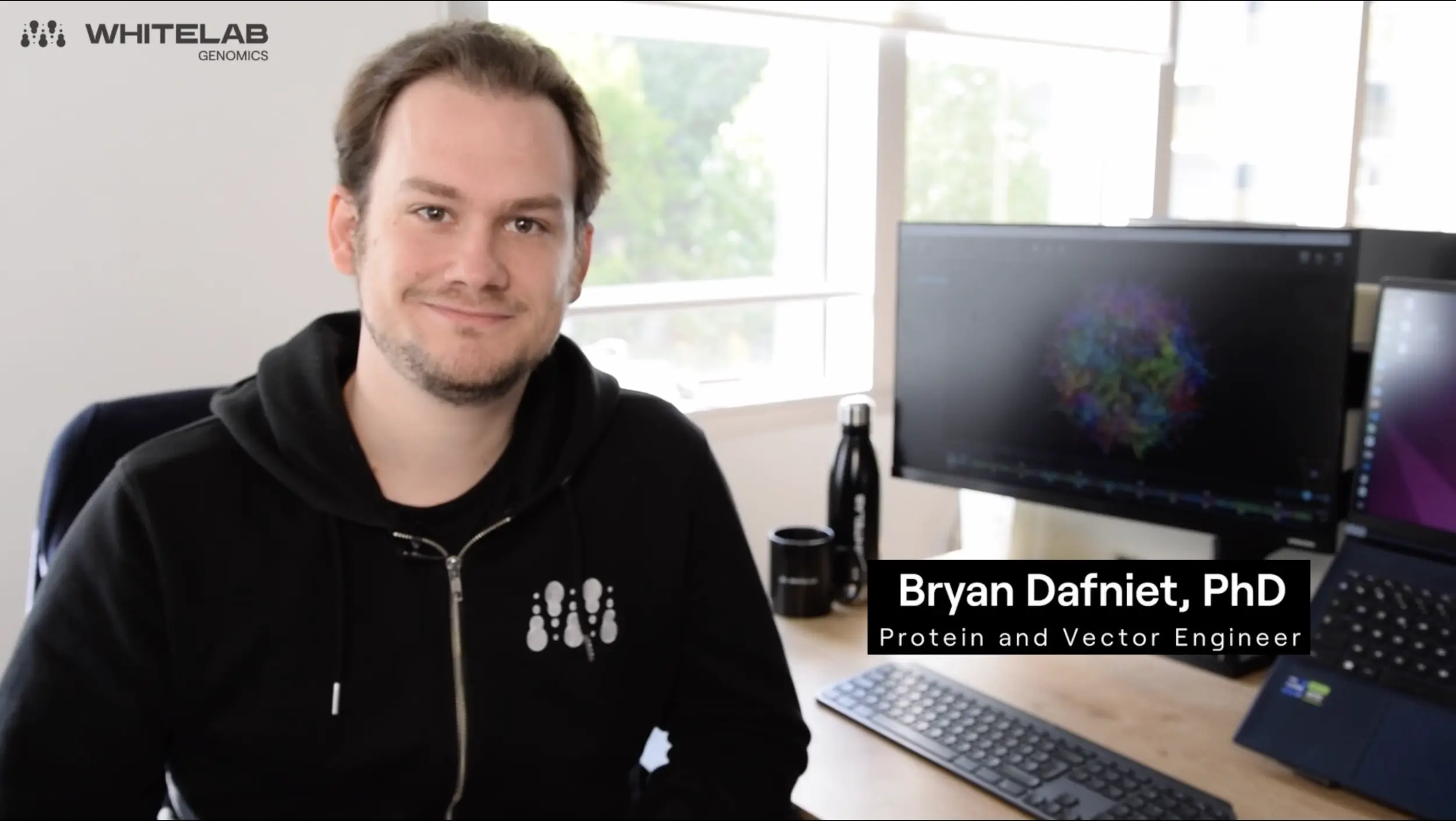
Revolutionizing Peptide Discovery: AI-Driven Drug Design Paving the Way for the Future of Cell & Gene Therapy
AMA1552 - Thursday, May 15, 5:30PM-7:00PM
Authors: C. Alliot, C. Colas, B. Dafniet, J. Maes, J-P. Buffet, P. Vidal, A. Murza, D. Del Bourgo, J.Cottineau, P-L. Boudreault, D. Serillon
WhiteLab Genomics, FUTURE4CARE, 8 rue Jean Antoine de Baif, 75013 Paris, France
Department of Pharmacology and Physiology, Faculty of Medicine and Health Sciences, Institut de Pharmacologie de Sherbrooke, Université de Sherbrooke, 3001, 12e Avenue Nord, J1H 5N4 Sherbrooke, Québec, Canada
Over the past decade, rational peptide design has gained significant momentum as a promising approach in drug discovery. Peptides are emerging as highly versatile therapeutic agents, offering unique advantages in targeting complex biological pathways. However, their design and development still represent a significant challenge due to their intrinsic structural flexibility and potential for unintended biological interactions, which can complicate therapeutic applications. Beyond their therapeutic potential, peptides may enhance gene delivery through various vector types and modifications. For Adeno-Associated Virus (AAVs) vectors, peptides can be inserted into their sequences to trigger better targeting. Peptides can also be conjugated as linear or cyclic forms to improve stability and binding of AAV vector or non-viral vector (e.g. Lipid Nanoparticles, LNP). Optimization opportunities include incorporating non-canonical amino acids to improve pharmacological properties. In this context, we apply a drug design protocol for the discovery of novel peptides towards a pivotal therapeutic target in oncology and immunotherapy. Despite extensive data availability, this receptor remains challenging for peptide-based drug design due to the atypical nature of the binding site: non-buried, without any specific pocket to be used as a basis for docking. However, the existing crystallized receptor structures provide a solid foundation for structure-based computational approaches, in addition to the availability of a reference peptide (Kd = 4.2nM), enabling competitive binding assays. WhiteLab Genomics’ AI-powered platform pre-screened tens of thousands of unique peptides extracted from our proprietary peptide library, employing a rational workflow: (i) Analysis of known binders to identify critical interaction motifs, (ii) Customization of computational tools tailored to receptor binding, (iii) Virtual screening of high-potential candidates, (iv) Structural optimization using SAR (Structure Activity Relationship) analysis, and (v) Peptides refinement through macrocyclization, sampling, and dynamic modeling. During the virtual screening phase, over 1,000 peptides were modeled in complex to predict binding affinity and assess virtual structure-activity relationships. Approximately 200 peptides were shortlisted based on predicted binding strength and compatibility with macrocyclization strategies. Using Surface Plasmon Resonance (SPR) assays, we evaluated the binding affinity of our peptides library and achieved hit rates of 8.3%. Notably, every hit demonstrated a higher binding affinity than the reference, underscoring the efficiency of our computational and experimental pipeline. Although no gold standard exists for both hit rate and hit definition, we considered the WhiteLab Genomics platform to have surpassed established field methodologies by defining hits as superior to the reference while still achieving an impressive hit rate. We are presenting an automated AI-driven platform for the discovery and optimization of peptide binders. This platform demonstrated exceptional performance, culminating in experimental validation of high-affinity binders (Kd) for a clinically relevant oncology target. These findings highlight the potential of AI-enhanced peptide design to drive the next generation of cell and gene therapy innovations.
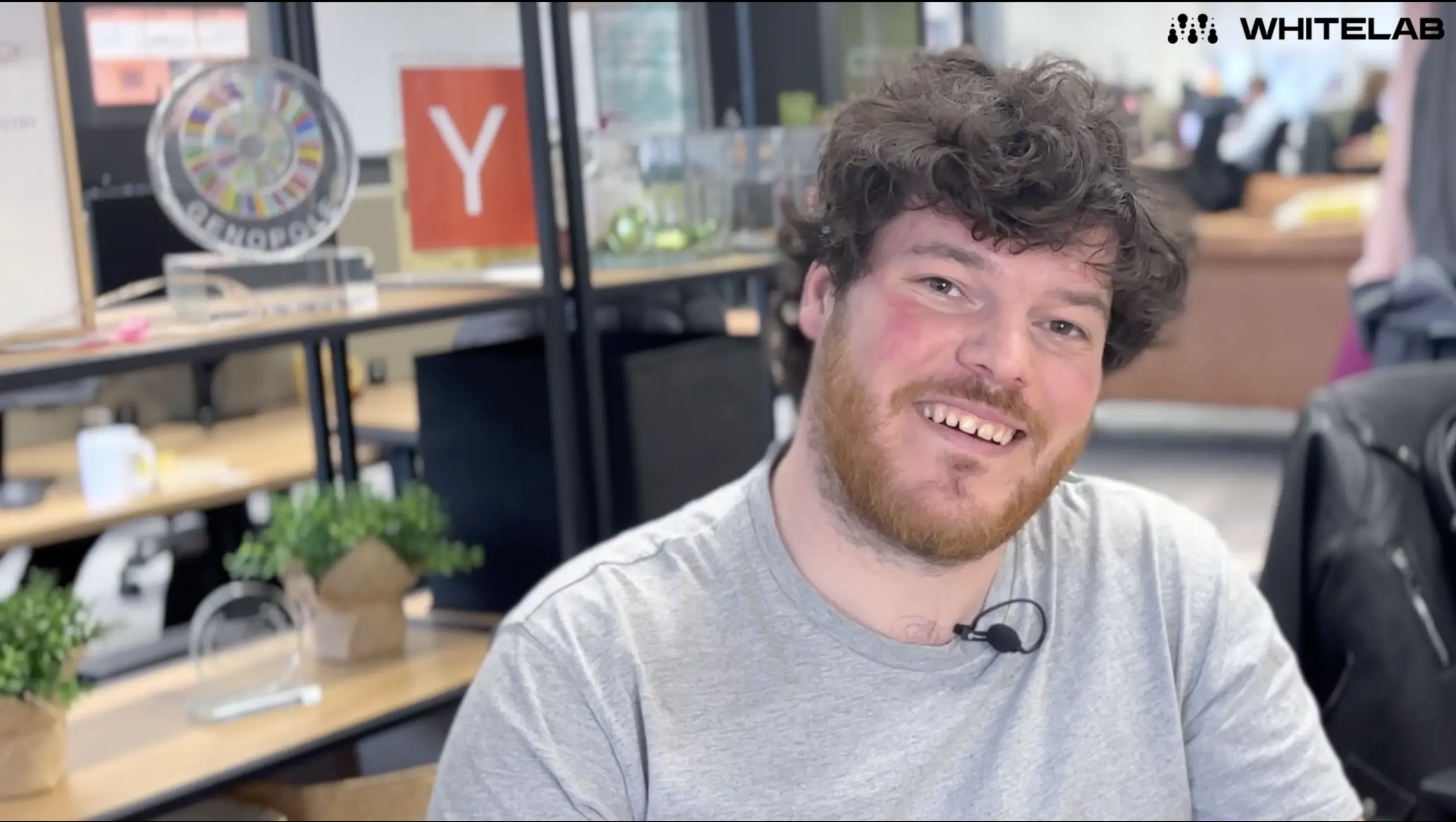